Publications
Teratology Primer, 3rd Edition
Can Computational Models Be Used to Assess the Developmental Toxicity of Environmental Exposures?
Thomas B. Knudsen, PhD, Developmental Systems Biologist, National Center for Computational Toxicology, US Environmental Protection Agency, Research Triangle Park NC 27711
Scope of the Problem
Environmental chemicals are natural and man-made compounds to which human populations are continually exposed as a matter of individual lifestyle, local geography, and community life. The Centers for Disease Control and Prevention (CDC) National Health and Nutrition Examination Survey (NHANES) surveys the U.S. population every two years to determine which chemicals get into people and at what concentrations [CDC, 2017]. NHANES has measured 308 environmental chemicals in blood or urine. Yet, we are all exposed to many more chemicals daily and lack data on developmental effects scenarios for most of the chemical landscape. The U.S. Environmental Protection Agency’s (EPA) Aggregated Toxicology Resource (ACToR) database indicates that developmental effects data are available for less than ~30% of 9,912 chemicals in commerce or of relevant environmental interest. And there are more chemicals synthesized on a daily basis. With tens of thousands of untested chemicals in the marketplace, the traditional animal-based paradigm does not meet the assessment needs.
In 2007, the National Research Council published Toxicity Testing in the 21st Century: A Vision and a Strategy (NRC, 2007). This report addressed the potential for automated high-throughput screening (HTS) and high-content screening (HCS) assays and technologies to identify chemically induced biological activity in human cells and to develop predictive models of in vivo biological response. This document ignited a shift in thinking from traditional animal endpoint-based testing to human pathway-based risk assessment paradigm. Since 2007 a surfeit of HTS/HCS data has fueled the paradigm shift and led to ‘big-data’ and integrative models for “encoding the toxicological blueprint of active substances that interact with living systems”, quoting Sturla and co-workers. Unprecedented amounts of data from genomic sciences, epidemiological studies, and HTS assays now provide the opportunity to profile the toxicological landscape. Impetus for this work is bolstered by the need to assess tens of thousands of environmental chemicals under European Registration, Evaluation, and Authorization of Chemicals (REACH) legislation and The Frank R. Lautenberg Chemical Safety for the 21st Century Act (Amended TSCA) in the United States. Under Amended TSCA, the EPA must prioritize and carry out studies to accelerate the development of scientifically valid test methods and strategies that reduce, refine, or replace the use of vertebrate animals and as well consider the impacts of chemical exposures on pregnant women and children as potentially exposed or susceptible populations.
Predictive Toxicology
As the primary source for regulatory developmental toxicity information, prenatal animal studies are used to characterize adverse pregnancy outcomes in rats and rabbits. The traditional paradigm is designed for a health protective effects assessment based on observation of fetal malformations in scenarios using relatively high doses. Approximately one in six chemicals show prenatal developmental toxicity under standard test conditions. For example, an initial assessment of adverse outcomes from standard prenatal developmental toxicity studies entered into the U.S. Environmental Protection Agency's (EPA) Toxicity Reference Database (ToxRefDB) revealed potential developmental toxicity for 53 of 283 (18.7%) environmental chemicals that had been tested in both pregnant rats and rabbits. If one of six chemical compounds were to evoke adverse developmental activity in a traditional testing paradigm, then prenatal exposure and developmental hazard data would be needed for some 13,800 of 83,000 environmental compounds falling under TSCA. A tiered testing strategy is therefore needed to filter large chemical inventories for follow-up studies. For example, an HTS assay that predicts teratogenicity in a human stem cell-based system in EPA's Toxicity Forecaster Database (ToxCastDB) reveals an exposure-based potential for 181 of 1065 (17.0%) environmental chemicals. Although the 17.0% positives predicted by a human stem cell response in vitro closely matches the 18.7% positives predicted by in vivo animal studies, only about one-third of those chemicals are predicted by both types of assays – human stem cells on the one hand and rat/rabbit studies on the other. Uncertainties exist even for compounds assessed by traditional animal models in translating results to human populations. Teratologists may turn to various kinds of in silico models to help reduce the uncertainties by providing information on exposure pathways and toxicological mechanisms.
Exposure Models
Environmental chemicals encompass a very wide diversity of chemical structures. Publicly available in silico models have been described with predictive application for developmental toxicity utilizing structure–activity relationships (SAR). Because the chemical structure is usually known, some developmental toxicants may be classified by a ‘decision tree’ for determining whether or not a chemical has receptor-binding properties and structural features consistent with related chemical structures known to have developmental or reproductive toxicity endpoints. The SAR decision tree could be used as a component of a tiered screening system to identify chemicals of potential concern or as a starting point for hypothesis-based testing.
Kinetic models predict what happens to the chemical once it enters the body: Absorption following oral intake, breathing dust, or contact with the skin; Distribution and partitioning to different body compartments; Metabolism to chemical forms that are more or less biologically active; and Excretion from the body. ADME factors vary by individual, species, and chemical based on the particular xenobiotic (foreign chemical) metabolizing enzymes in the organ. Kinetic models can predict internal dose to a human embryo when the mother is exposed to low concentrations of the chemical for prolonged periods of time. Such information is necessary to determine the range of exposures that may be relevant for potential human toxicity. These models do not, however, address mechanisms.
Adverse Outcome Pathways (AOPs)
Teratogenic mechanisms may be modeled conceptually as an adverse outcome pathway (AOP) (Figure 1). An AOP starts with a distinct molecular initiating event (MIE) and describes the sequelae of key events (KEs) that culminate in a measureable adverse outcome (AO) relevant to effects assessment. An AOP says “here is a biological perturbation that can lead to a specific adverse outcome, and here is how we think it happens”. A particular malformation, such as thalidomide-induced phocomelia or alcohol-induced craniofacial defects, is constructed from weight-of-evidence supporting MIE targets and KE relationships for developmental toxicity and from biological understanding of developmental pathways and processes.
Figure 1. Principles for building an AOP. (1) AOPs are not chemical-specific, but rather based on biological motifs of failure. (2) AOPs are modular, with the individual relationships based on weight of evidence from the extant literature. (3) Individual AOPs are a pragmatic simplification and are constructed as a linearized sequence of biology. (4) AOP networks are, in most cases, the functional unit of prediction. (5) AOPs are living documents and evolve as knowledge grows. A knowledgebase holds the compendium of AOPs with demonstrated relevance to the mode-of-action for specific chemicals and may be accessed from the internet at https://AOPwiki.org. Figure courtesy of D Villeneuve, US EPA.

In 2000, the National Academy of Sciences issued a report advocating the use of detailed knowledge about cell signaling pathways to help elucidate mechanisms in developmental toxicity. That report summarized a listing of molecules mediating cell signaling pathways across species and developmental processes as well as the qualitative relationships between the molecular components in 17 cell–cell signaling pathways and two stress response pathways having conserved roles in animal development. Although these pathways provide a means to understand how cellular decisions are timed, controlled, and orchestrated during embryogenesis, it still remains to be determined how they are wired into AOPs for prenatal developmental toxicity. For example, cyclopamine, a natural plant product consumed by foraging sheep, blocks cholesterol esterification of the sonic hedgehog protein (SHH) as an MIE, leading to a disruption of SHH signaling that patterns forebrain development and in turn formation of a single midline eye (cyclopia) as an adverse outcome.
High-throughput screening
Automated technologies originally developed for pharmaceutical screens are now being used to profile cellular effects of thousands of chemical compounds in commerce or potentially entering the environment. In a drug development paradigm, chemical libraries containing thousands of unique structures may be rapidly screened for target biological activity against specific cell lines in a high-throughput mode. Active compounds may be used as leads to design compounds that would have the desired in vivo efficacy; they are then evaluated in animal tests, clinical trials, and post-marketing surveillance. For example, the National Center for Advancing Translational Sciences of the NIH has the capacity for automated high-throughput screening of 100-200K compound libraries across dozens of in vitro assays.
Applying the pharmaceutical high-throughput screening paradigm to toxicity testing turns the drug discovery process upside down (Figure 2). Environmental chemicals of unknown or suspected human toxicity may be tested through hundreds of in vitro assays. Computers are then used to look for patterns of biological activity across the assay portfolio and chemical library. These profiles may be compared with reference compounds of well-characterized biological activity or interrogated in different ways, looking for in vitro signatures that are potentially diagnostic of in vivo toxicities. For example, EPA’s ToxCast™ project is providing high-throughput screening data on ~2000 chemicals for proof-of-concept on ~600 assays. The broader “Toxicity Testing for the 21st century” (Tox21) federal consortium is ramping up to test ~8000 chemicals of importance to commerce and the environment [http://www.epa.gov/ncct/toxcast/]. Alternative assays, such as embryonic stem cells and free-living zebrafish embryos, can be used to rapidly test chemical effects in systems undergoing morphogenesis, growth, and differentiation.
Figure 2. HTS paradigm for pharma (top) and industrial chemicals (bottom). Databases and computer models that track exposure-disease correlations, as well newer technologies that permit ‘high-throughput screening’ of the molecular and cellular pathways of toxicity can help to fill in our knowledge gap with respect to developmental toxicity of environmental chemicals.

‘Big-Data Analytics’
The older concept of one chemical, one target has become outdated with the realization that environmental chemicals, as well as many pharmaceuticals, have a range of potential molecular targets that may engage at different concentrations, cell types, lifestages, or physiological states. EPA’s ‘ToxCast Dashboard’ helps users examine high-throughput assay data to inform chemical safety decisions. To date, the dashboard has data on over 9000 chemicals and information from more than 1000 high-throughput assay components [https://www.epa.gov/chemical-research/toxcast-dashboard] including nearly a million concentration-response curves that can be mined for complex relationships between chemical structures, molecular targets, and cellular responses. Users of the dashboard can explore the dataset from a chemical or an assay viewpoint. After selecting the chemicals and assays of interest he or she can then explore the biological activity for the chemical-assay combinations.
New science can emerge from analysis of the surfeit of HTS data and information now available for thousands of chemicals in the ToxCast/Tox21 databases. But, a practical need arises to tie these data in some way to formal biological understanding. This effort is part of a bigger picture, ‘big-data’ analytics: looking for hidden correlations that are difficult to extract from individual association in large sets of unsorted, noisy data. Challenges facing this type of predictive modeling include correlating in vitro concentration-response with internal dose-response kinetics, understanding how in vitro bioactivity profiles can be extrapolated from one cell-type or technology to another, and linking individual targets of in vitro bioactivity into pathways of toxicity. These challenges cycle back to a need for computational models that merge high-throughput screening data with biological knowledge. A deep understanding of embryological development, intuition for new technologies and approaches to the study of birth defects, and a concern for environmental influences on human development are all driving the new vision on where environmental health protection needs to go. Computational biology is uniquely positioned to advance this science and technology. For developmental toxicology, this means making sense of ‘big-data’ on a broader scale without falling prey to a meaningless mass of interconnected data linkages.
Computational Biology
The explanatory and predictive power of simple informal models has its limits. Development functions through ‘systems dynamics’. As the biological complexity becomes clearer to us, a more quantitative approach has become essential for predicting developmental toxicity. Computational models can help translate genetic signals and responses at the subcellular level into multicellular networks that drive morphological development at the organ level. Multicellular Agent-Based Models (ABMs) that execute a morphogenetic series of events can bridge the gap between in vitro profiling and in vivo response at different concentrations of chemical. The general idea of an ABM is to represent the ‘cell’ of an embryonic tissue as an ‘agent’, i.e. the smallest fundamental unit capable of an autonomous decision. Individual agents and their interactions are coded into modeling software such as CompuCell3D.org based on our understanding of cellular signals, responses, and behaviors. Each simulated cell, like a biological cell, processes local cues from its environment and behaves according to its own blueprint or history. Running a multicellular simulation enables cell-cell interactions in a shared microenvironment that includes an array of physiological signals that mediate cell-cell communication. The collective multicellular behavior results in some emergent (non-coded) property that can be recorded, analyzed, and interpreted in ways similar to experimental teratology.
Rendering an ABM is ideal for predictive toxicology because it integrates information across different biological scales including molecular information (internal clocks, biochemical gradients, and gene regulatory networks), cellular properties (growth, adhesion, and differentiation), and tissue-level properties (homeostasis, morphogenesis, and repair). These approaches have successfully been applied to reconstruct a morphogenetic series of events and perturb them as an approximation. See developmental toxicity models for the vasculature, genital tubercle, and palate for examples (Figure 3).
Figure 3. Cell agent-based model of palatal development. Made practical by computational advances and quantitative data from HTS, these dynamical models formally reconstruct tissue development cell-by-cell, interaction-by-interaction. The model shown here recapitulates the outgrowth and fusion of paired palatal shelves in the embryo to form a uniform rudiment of the hard palate. Introducing perturbations to key genetic signals and responses gives rise to in silico phenotypes or ‘cybermorphs’. By introducing ToxCast lesions into the model, the computer simulates lesion propagation through various developmental trajectories and returns a quantitative prediction of cleft palate [Hutson et al., 2017].
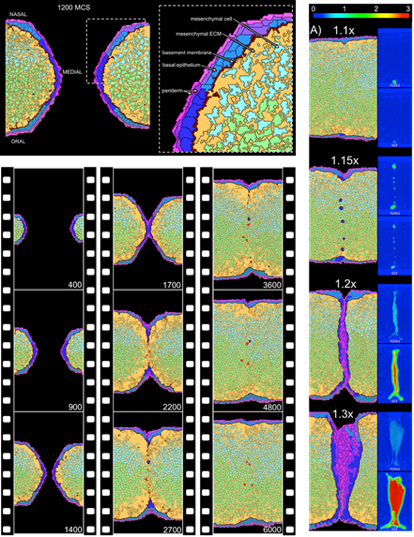
So, to return to the original question, “Can Computational Models Be Used To Assess The Developmental Toxicity of Environmental Exposures?” Certainly they can operationalize in vitro data from HTS testing platforms. Much effort is being expended today to realize this goal for the future. EPA’s Virtual Embryo is building and testing computational (in silico) models that may make high-throughput screening data useful in a quantitative risk assessment of developmental toxicity [http://www.epa.gov/ncct/v-Embryo/].
Disclaimer: The United States Environmental Protection Agency through its Office of Research and Development funded and managed the research described and this paper has been subjected to Agency review and approved for publication. Reference to commercial products or services does not constitute endorsement.
REFERENCES
Centers for Disease Control and Prevention (CDC) (2017) Fourth National Report on Human Exposure to Environmental Chemicals, 2009, Updated Tables, January 2017. [http://www.cdc.gov/exposurereport].
Collins FS, Gray GM and Bucher JR (2008) Transforming environmental health protection. Science 319: 906-907.
Engler AJ, Humbert PO, Wehrle-Haller B and Weaver (2009) Multiscale modeling of form and function. Science 324: 208-212.
Hutson MS, Leung MCK, Sipes NS, Baker NC and Knudsen TB (2017) Computer modeling of palatal fusion and simulation of toxicant disruption. Chem Res Toxicol 30: 965-979.
Judson RS, Houck KA, Kavlock RJ, Knudsen TB, Martin MT, Mortensen HM, Reif DM, Richard AM, Rotroff DM, Shah I and Dix DJ (2010) Predictive in vitro screening of environmental chemicals – the ToxCast project. Environ Hlth Persp 118: 485-492.
Judson R, Houck K, Martin M, Richard AM, Knudsen TB, Shah I, Little S, Wambaugh J, Setzer RW, Kothiya P, Phuong J, Filer D, Smith D, Reif D, Rotroff D, Kleinstreuer N, Sipes N, Xia M, Huang R, Crofton K and Thomas RS (2016) Analysis of the Effects of Cell Stress and Cytotoxicity on In Vitro Assay Activity in the ToxCast Dataset. Tox Sci 152: 323–329.
Kleinstreuer N, Dix D, Rountree M, Baker N, Sipes N, Reif D, Spencer R and Knudsen T (2013) A computational model predicting disruption of blood vessel development. PLoS Comput Biol. 9: e1002996.
Knudsen TB, Martin MT, Kavlock RJ, Judson RS, Dix DJ and Singh AV (2009) Profiling the activity of environmental chemicals in prenatal developmental toxicity studies using the U.S. EPA’s ToxRefDB. Reprod Toxicol 28: 209-219.
Knudsen TB and Kavlock RJ (2008) Comparative bioinformatics and computational toxicology. In: Developmental Toxicology volume 3, Target Organ Toxicology Series. (B Abbott and D Hansen, editors) New York: Taylor and Francis, pp 311-360.
Leung MCK, Hutson MS, Seifert AW, Spencer RW and Knudsen TB (2016) Computational modeling and simulation of genital tubercle development. Reprod Toxicol 64: 151-161.
Knudsen TB, Zurlinden TJ, Saili KS, Palmer J and Baker NC (2018) Profiling the ToxCast library with a pluripotent human (H9) embryonic stem cell assay. Abstract in press, Society of Toxicology, San Antonio TX, March 2018.
National Research Council (2000) “Scientific Frontiers in Developmental Toxicology and Risk Assessment”. National Academy Press (Washington, DC). http://www.nap.edu/books/0309070864/html/
National Research Council (2007) Toxicity Testing in the 21st Century: A Vision and a Strategy. The National Academies Press, Washington, DC, 196 pages.
Richard AM, Judson RS, Houck KA, Grulke CM, Volarath P, Thillainadarajah I, Yang C, Rathman J, Martin MT, Wambaugh JF, Knudsen TB, Kancherla J, Mansouri K, Patlewicz G, Williams AJ, Little SB, Crofton KM and Thomas RS (2016) ToxCast Chemical Landscape: Paving the Road to 21st Century Toxicology. Chem Res Toxicol 29: 1225-1251.
Sturla SJ, Boobis AR, Fitzgerald RE, Hoeng J, Kavlock RJ, Schirmer K, Whelan M, Wilks MF and Peitsch MC (2014) Systems toxicology: from basic research to risk assessment. Chem Res Toxicol 27: 314-329.
Villeneuve DL, Crump D, Garcia-Reyero N, Hecker M, Hutchinson TH, LaLone CA, Landesmann B, Lettieri T, Munn S, Nepelska M, Ottinger MA, Vergauwen L, and Whelan M (2014) Toxicol Sci 142: 312–320.
Wu S, Fisher J, Naciff J, Laufersweiler M, Lester C, Daston G, and Blackburn K (2013) Chemical Research in Toxicology 26: 1840–1861.